Harnessing the Power of Labeling Tool Machine Learning for Business Success
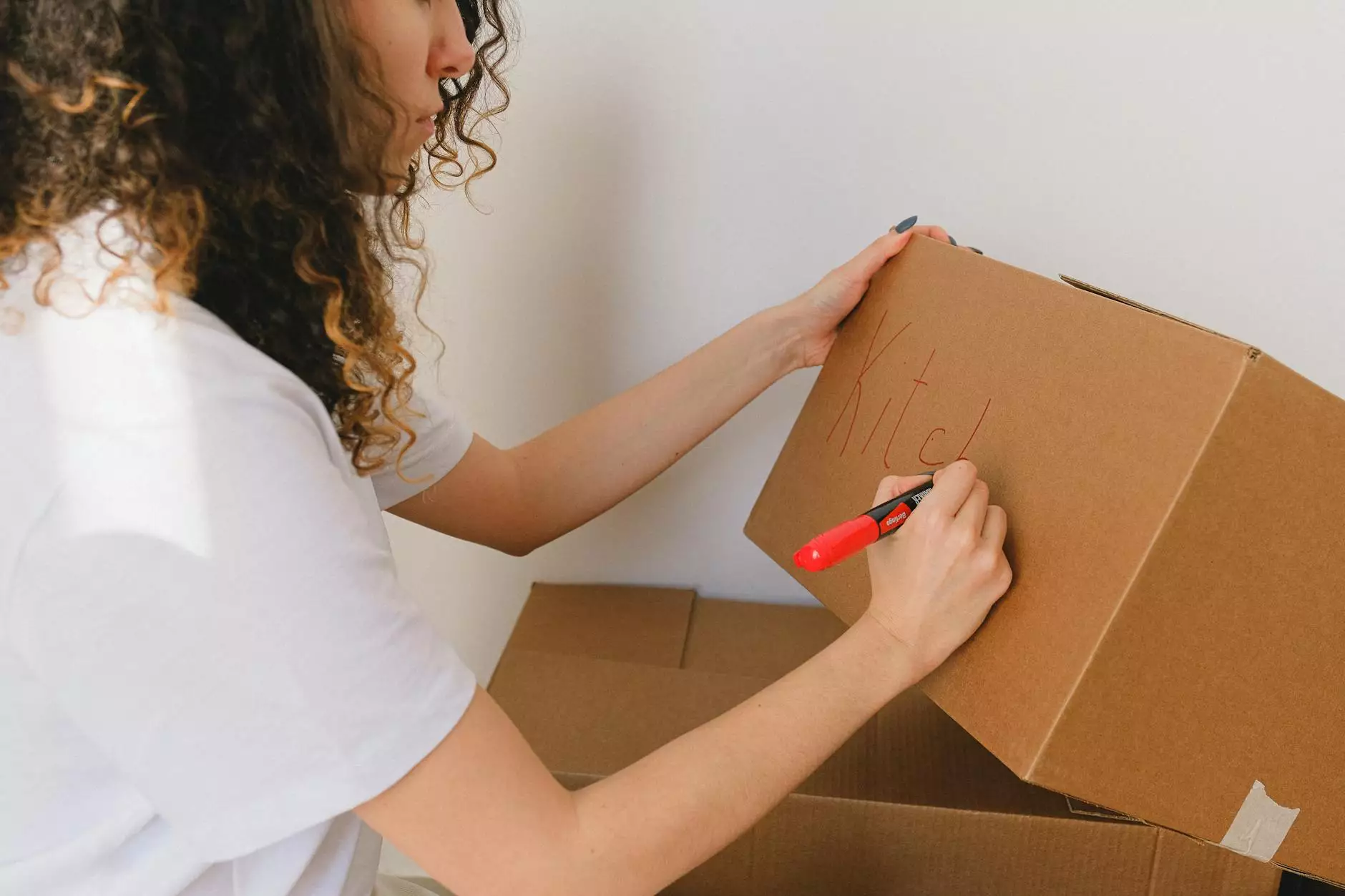
In today’s fast-paced and technology-driven world, businesses are increasingly turning to advanced solutions to keep up with the demands of data management and analysis. One of the most groundbreaking innovations in this field is the labeling tool machine learning. This powerful tool not only enhances productivity but also adds a layer of accuracy and efficiency that is crucial for modern enterprises. In this article, we will explore the significance of labeling tools in machine learning, their applications, benefits, and how businesses can leverage these tools to maintain a competitive edge.
Understanding Labeling Tool Machine Learning
The core of machine learning is data. In order to train algorithms, you must have high-quality data that is accurately labeled. Essentially, labeling tool machine learning involves the process of annotating data, which can include images, text, videos, and more. Each piece of data is given a tag or label, which enables algorithms to learn from these labeled examples and make predictions or classifications.
What is Data Annotation?
Data annotation is the process of adding meaningful labels to raw data. It can take several forms, including:
- Image Annotation: Identifying and labeling objects in images.
- Text Annotation: Tagging parts of text for sentiment analysis, named entity recognition, etc.
- Video Annotation: Labeling video content for action recognition or object tracking.
By applying machine learning techniques to these tasks, businesses can automate the daunting and time-consuming data annotation process, thereby freeing up human resources for more strategic tasks.
The Importance of Accurate Data Annotation
Accurate data annotation is vital for the success of machine learning models. Poorly labeled data can lead to flawed predictions and ultimately compromise the quality of business decisions. Here are a few reasons why businesses should invest in labeling tools:
- Enhanced Model Accuracy: Well-labeled data improves the performance and accuracy of machine learning models.
- Time Efficiency: Automating the labeling process reduces the time taken to prepare data for analysis.
- Cost-Effectiveness: Reduces the need for extensive manual labor, translating into lower operational costs.
How Keylabs.ai Stands Out in the Data Annotation Space
Keylabs.ai provides state-of-the-art data annotation tools and platforms that leverage labeling tool machine learning to empower businesses. Their solutions are designed to handle vast datasets with ease and deliver high-quality annotations that meet industry standards.
Features of Keylabs.ai Annotation Tools
The tools offered by Keylabs.ai come with a plethora of features that make them indispensable for businesses looking to harness the power of labeling tool machine learning:
- Scalability: Easily scale your annotation efforts as your data volume increases.
- Customization: Tailor the annotation tools to meet the specific needs of your business.
- Collaboration: Facilitate seamless collaboration with teams across the globe.
- Quality Assurance: Integrated QA processes ensure high-quality annotations.
Applications of Labeling Tool Machine Learning in Various Industries
Healthcare
In the healthcare industry, accurate data labeling is crucial for developing predictive models for disease diagnosis and patient care. By using labeling tool machine learning, medical researchers and practitioners can analyze medical images, patient records, and more, helping them make informed decisions.
Autonomous Vehicles
Autonomous vehicles rely heavily on labeled data for training their AI systems to recognize and respond to various objects on the road. Labeling tools are essential in providing high-quality datasets that enhance the safety and reliability of these vehicles.
Retail
In retail, machine learning models use customer data to provide personalized experiences. Properly labeled data allows these businesses to analyze consumer behavior effectively, optimizing marketing strategies and product offerings.
Challenges in Data Annotation
Despite its advantages, data annotation comes with challenges that businesses must be aware of:
- Cost of Labor: Manual data annotation can be expensive and time-consuming.
- Quality Control: Maintaining the quality of annotations can be difficult, especially without the right tools and processes.
- Scalability Issues: As data grows, the annotation process must scale efficiently to keep up.
Overcoming Challenges with Automation
Technological advancements in labeling tool machine learning automate many processes involved in data annotation, effectively overcoming the challenges mentioned above. Here’s how automation transforms the landscape:
- Reducing Labor Costs: Automation minimizes the reliance on manual annotations, significantly reducing costs.
- Improving Consistency: Automated tools provide consistent and repeatable outcomes for data labeling.
- Speeding Up Processes: Automated annotation can process large datasets in a fraction of the time it takes for manual labeling.
Best Practices for Implementing Labeling Tool Machine Learning
To maximize the benefits of labeling tool machine learning, businesses should consider the following best practices:
- Define Clear Objectives: Set specific goals regarding what you need to achieve with data annotation.
- Choose the Right Tools: Select annotation tools that fit your data types and business requirements.
- Invest in Quality Assurance: Implement QA processes to ensure the accuracy of labeled data.
- Train Your Team: Provide training on using annotation tools effectively to maximize productivity.
Future of Labeling Tool Machine Learning
The future of labeling tool machine learning looks promising, with emerging technologies like Artificial Intelligence (AI) and Natural Language Processing (NLP) set to revolutionize data annotation:
- Self-Learning Systems: Future tools may evolve to label data autonomously, refining their accuracy over time.
- Integration with Other Technologies: Combining data annotation tools with IoT and blockchain can provide enhanced security and traceability.
- Cross-Industry Applications: As AI continues to advance, its applications across diverse industries will keep expanding.
Conclusion
The integration of labeling tool machine learning into business processes signifies a monumental shift towards intelligent data management. With platforms like Keylabs.ai, organizations can not only enhance their operational efficiency but also gain invaluable insights that drive growth and innovation. As technology evolves, embracing these tools becomes imperative for any business aiming to thrive in the digital age.
Ultimately, ensuring the quality of data through robust labeling mechanisms will lay the foundation for successful machine learning initiatives — empowering businesses to harness the full potential of their data in a competitive marketplace.